Harnessing 'Big Data'
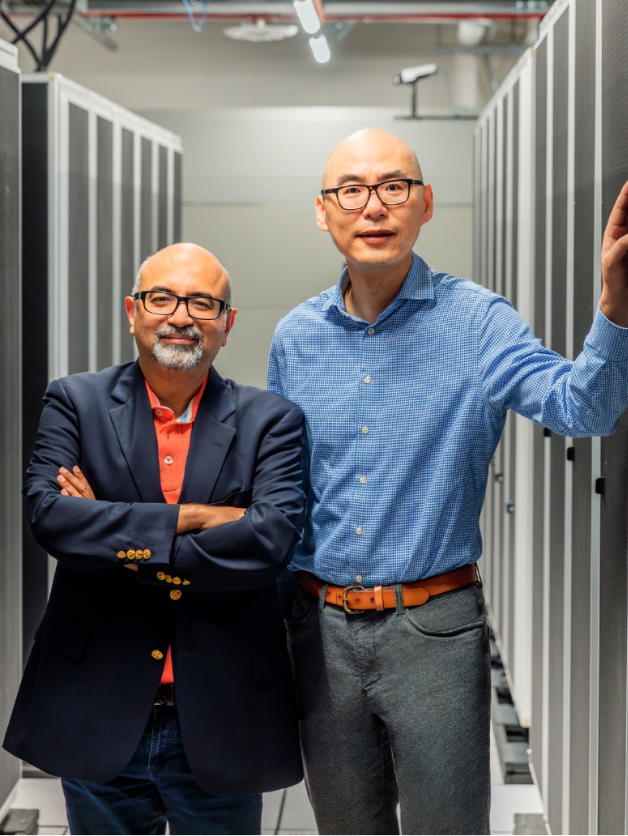
In 1964, when the UCLA Fielding School of Public Health was in its third year, IBM released System/360, a mainframe computer that would dominate the market through the 1970s. The largest of the machines held 8 megabytes of memory. From there, the growth was exponential. In 1980, the company’s refrigerator-sized IBM 3380 was the first with a gigabyte of hard-drive capacity; today, the smartphones we carry in our pockets have more than 100 times that. And in the era of what is often referred to as “big data,” researchers at UCLA Fielding and beyond can tap into the limitless possibilities presented by high-powered computing, machine learning, and the explosion of information from wide-ranging sources to glean insights and draw conclusions that can improve the health of populations.
What hasn’t changed in the school’s more than six decades is the vital role of biostatisticians in harnessing the data and calculating uncertainties to ensure that scientifically sound conclusions are reached. UCLA Fielding’s new Master of Data Science in Health (MDSH) program continues the school’s tradition of educating students to meet the ever-evolving demands in the field of biostatistics and data science. Dr. Sudipto Banerjee (image, left), FSPH’s senior associate dean for academic programs, is a professor of biostatistics who served as chair of the department for nine years, and is an expert on Bayesian hierarchical modeling and inference for spatially oriented data. Dr. Hua Zhou (image, right), FSPH professor of biostatistics and director of the new MDSH program, who joined the faculty in 2015, is an expert in big data, machine learning, and applications in areas such as genomics, electronic health records, and neuroimaging.
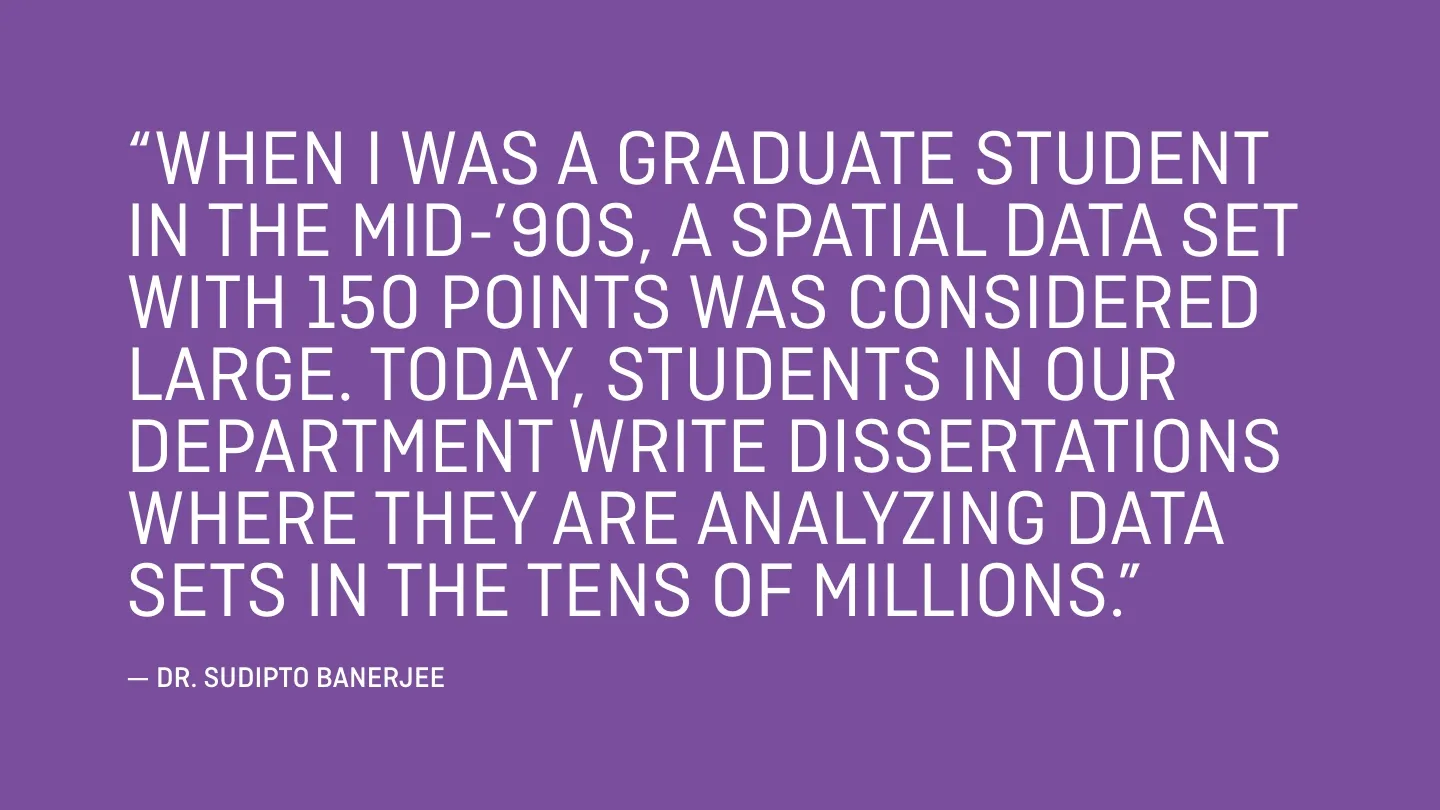
HOW HAS THE FIELD OF DATA SCIENCE IN PUBLIC HEALTH EVOLVED OVER THE COURSE OF YOUR CAREER, PARTICULARLY AS REFLECTED IN THE WORK OF BIOSTATISTICIANS AT UCLA FIELDING?
SUDIPTO BANERJEE: It’s been a tsunami of change. When I was a graduate student in the mid-’90s, a spatial data set with 150 points was considered large. Today, students in our department write dissertations where they are analyzing data sets in the tens of millions. In my years as a student, we operated mostly on mainframe computers. Now, to be at the cutting edge of research, we need to harness the most powerful computing resources available to us. And in that regard, our department truly stands out when it comes to fundamental statistical computing research and big-data analysis in diverse fields. We have several faculty members who are undisputed leaders. And that leadership can be traced to the origins of our school. One of the most widely used statistical modeling and data analysis software programs of its time, BMDP [Bio-Medical Data Package], was developed by two of our earliest biostatistics faculty leaders, Frank Massey Jr. and Wilfrid Dixon. Since then, we have had many scholars who made tremendous contributions to the development of the field, including our former dean, Abdelmonem A. Afifi, in multivariate analysis.
HOW HAVE THE TOPICS BIOSTATISTICIANS TAKE ON CHANGED OVER THE YEARS?
SB: We are increasingly required to analyze data in very complex scientific settings. Our faculty have strong collaborations with the medical school, working on studies involving genetics and genomics, the analysis of neuroimages, electronic health records, and biobank research. In my domain, we use geographic information systems to analyze population health data over space and time. As the field of public health has evolved, biostatisticians are looking at, for example, understanding the impact of climate change on health. To do that, we have to relate variables of climate science with health science variables. And so, in that regard, a modern biostatistician needs a good understanding of models in climate science as well as models in public health.
HUA ZHOU: That’s a major difference between biostatistics and statistics — biostatisticians spend a lot of effort trying to understand the problem and the special characteristics in the data related to that problem, then designing statistical modeling strategy, based on that understanding, for the analysis. And it’s not only in the analysis stage where biostatisticians are important. It’s also at the stage of study design: What’s optimal to minimize cost, given budget restrictions, while maximizing impact? And after you collect the data, what’s the best method for analyzing it?
WHAT IS MEANT BY “BIG DATA,” AND HOW IS IT CHANGING THE FIELD?
SB: The most basic definition is that you are trying to analyze a data set that is too massive to be stored in your hard drive. Traditional statistical methods are simply unable to analyze these massive data sets because of memory requirements. But just as important as the size is the question you’re asking of that data set. If you’re just calculating an average from a trillion numbers, that can be done, even in fairly modest computing architectures. But if it involves understanding dependencies and relationships among all these variables, it becomes a much more complicated problem that requires special methods.
HZ: We talk about the V’s of big data. The obvious one is volume, but there are others. A second is variety. It’s not just numbers. You can have image data, or text data — using blogs or social media to analyze trends in the COVID-19 pandemic, for example. A third is velocity. With learning or streaming data, like for a self-driving car, sensors are taking pictures every second and you need to make a dynamic decision. Smart devices, such as iPhones and Fitbits, are collecting data in real time, and we’re seeing more applications for health. And a fourth V is veracity. With generative AI and deep learning, data can be faked. Statisticians have long studied uncertainty in the data, and that’s especially relevant now
HOW IS BIG DATA CHANGING THE SKILLS DEMANDED OF BIOSTATISTICIANS, AND THE WAY UCLA FIELDING EDUCATES THEM?
HZ: When I was a student, the textbooks used data sets with a couple of dozen individuals getting measured on five or six variables. You could go out to the job market — say, in the pharmaceutical industry — and get a position as a statistician without much programming experience. You would just write a statistical analysis plan, someone else would program for you, and then you would interpret the results. Today, when you get a job as a data scientist, the expectation is that you know machine learning and statistical theory, and that you will be able to program and deploy the method to a massive data setting. That’s one of the reasons we revamped our curriculum and established the new Master of Data Science in Health degree program. There’s a gap between classical training and the current demand for expertise in meeting these big-data challenges.
WHAT IS IT THAT CONTINUES TO MAKE THE BIOSTATISTICIAN’S ROLE IN PUBLIC HEALTH SO ESSENTIAL?
SB: In my opinion, the most important aspect of our expertise is the quantification of risks or uncertainties. We protect against spurious and inaccurate scientific conclusions, which offers insurance against, or even prevents, wrong decisions. We can all agree that weather prediction has improved substantially over the years. A major reason is that it has gone from what was purely a physical science exercise to one that brings together the mathematical models from the physical sciences with statistical models. What can go wrong when sound statistical analysis isn’t incorporated in weather prediction? You might carry an umbrella more than you need to, or not carry one when you need one. But imagine if we were completely off on the number of people we thought would be infected from COVID. Early in the COVID-19 pandemic, statisticians were at the forefront of conducting analyses so that the projection of how this pandemic was going to evolve, and the policies formulated based on those projections, were done in a robust and statistically sound way. This had a massive impact on what policies would be framed amid the pandemic.
WHAT MAKES YOU HOPEFUL, AS A BIOSTATISTICIAN, ABOUT PUBLIC HEALTH’S ABILITY TO TACKLE THE DAUNTING CHALLENGES AHEAD?
HZ: I’m excited to be working in such a burgeoning field, where there are so many open questions and challenges. What makes me happy is seeing our students learning big data techniques and using them to do very impactful research.
SB: I don’t think there has been a time in the history of our profession when data-centric technologies and discoveries have been more important. And when I see my colleagues — incredibly talented, gifted intellectuals and scholars — working to address these challenges, I am very confident of a bright future. With so many gifted, energetic, and skilled young individuals entering this profession, how can I not be optimistic?
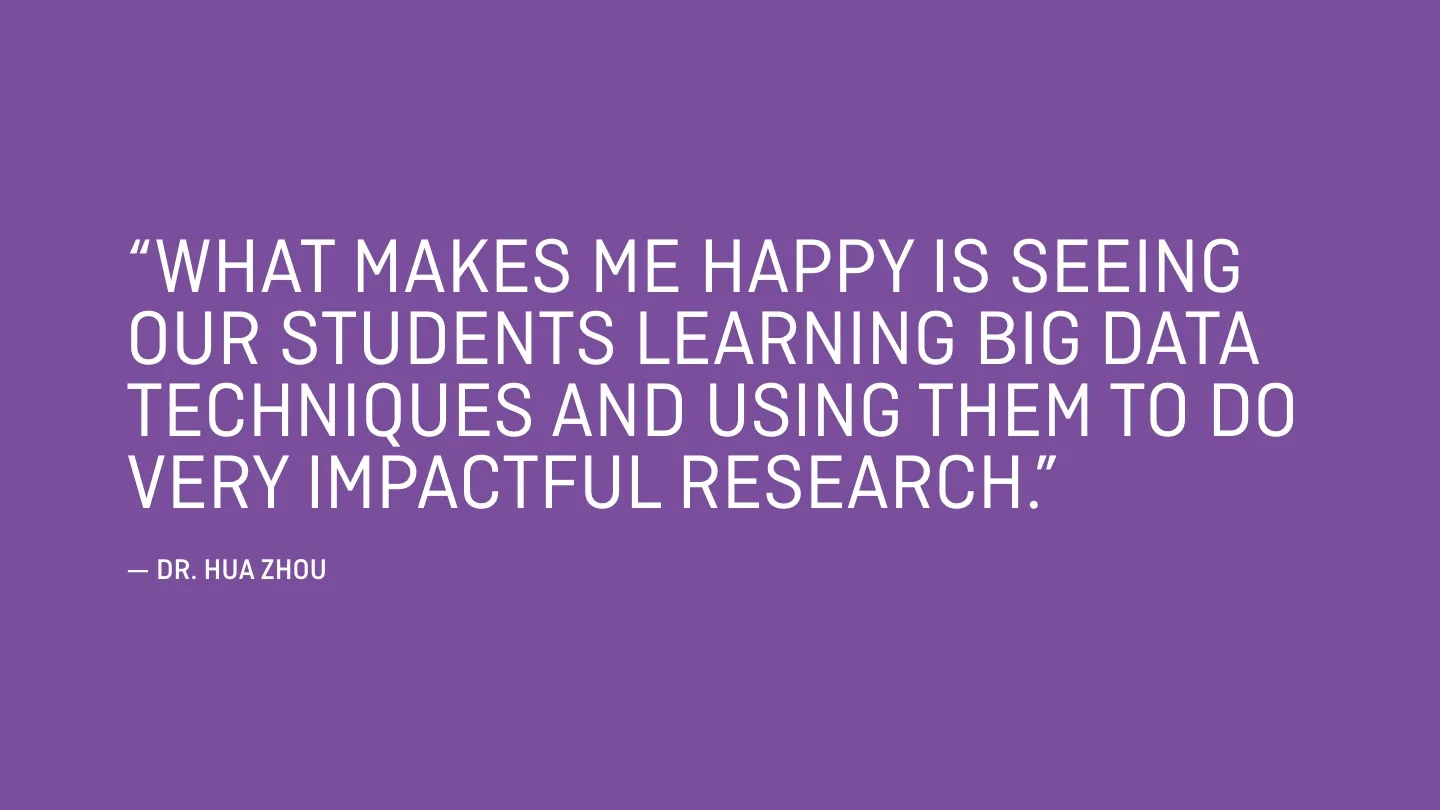
Faculty Referenced in this Article
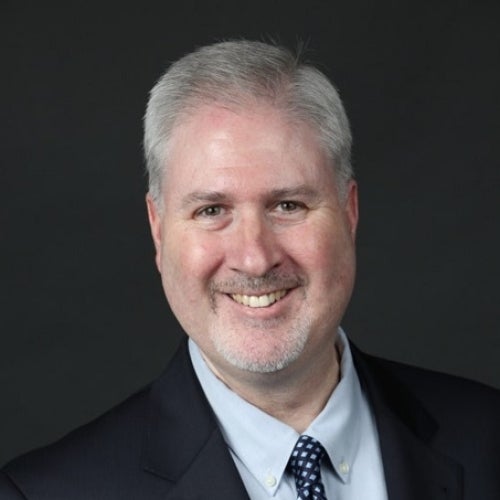
Automated and accessible artificial intelligence methods and software for biomedical data science.