Lancet paper shows most popular hypertension drug isn’t most effective, per OHDSI’s LEGEND study
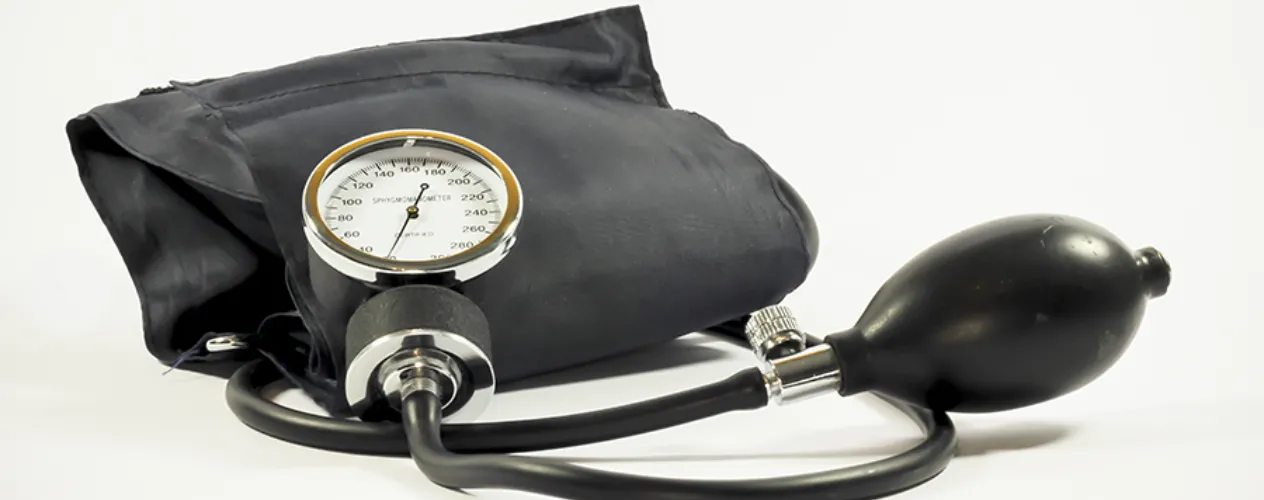
Thiazide diuretics demonstrate better effectiveness and cause fewer side effects than ACE inhibitors as first-line antihypertensive drugs, according to a report published Oct. 24 in The Lancet. The study factors insurance claim data and electronic health records from 4.9 million patients across nine observational databases, making it the most comprehensive one ever on first-line antihypertensives, and it provides additional context to the 2017 guidelines for high blood pressure treatment developed by the American College of Cardiology (ACC) and American Heart Association (AHA).
Collaborators in the Observational Health Data Sciences and Informatics (OHDSI) network produced the paper “Comprehensive comparative effectiveness and safety of first-line antihypertensive drug classes: a systematic, multinational, large-scale analysis” as part of the collaborative’s ongoing Large-Scale Evidence Generation and Evaluation across a Network of Databases (LEGEND) project, which applies high-level analytics to perform observational research on hundreds of millions of patient records within OHDSI’s international database network.
OHDSI researchers believe LEGEND will continue to significantly enhance how real-world evidence is used to study important healthcare questions that impact millions of patients worldwide.
First-Line Thiazide Diuretic Users Experience 15% Fewer Adverse Cardiovascular Outcomes Than ACE Inhibitor Users
The 2017 ACC/AHA guidelines on antihypertensives recommend initiating hypertension (high blood pressure) treatment with prescription medications from any of five drug classes, including both thiazides and ACE inhibitors. Within the LEGEND project, ACE inhibitors produced both worse cardiovascular outcomes and worse side effects than thiazides.
First-line thiazide new-users experienced three major medical outcomes (heart attack, hospitalization for heart failure, and stroke) at an approximate 15% lower event rate than those who began treatment with an ACE inhibitor. Furthermore, among potential side effects associated with first-line hypertensive drugs, ACE inhibitor new-users experienced a higher rate of 19 potential side effects — and a lower rate of 2 — than thiazide diuretic new-users.
In spite of these differences, the majority of patients from this study who initiated treatment were prescribed ACE inhibitors (48%) over thiazides (17%); the results, however, indicate that over 3,100 major cardiovascular events could potentially have been avoided had those approximately 2.4 million ACE inhibitor new-users chosen a thiazide diuretic instead.
Filling The Evidence Gaps
“The LEGEND project attempts to fill the evidence gaps in treatment choices that randomized controlled trials (RCTs) leave unanswered,” said lead author Dr. Marc A. Suchard, professor of biostatistics at the UCLA Fielding School of Public Health, and biomathematics and human genetics at UCLA. “We were able to compare all antihypertensive drug classes against each other at a massive scale and in a transparent and reproducible manner to study what patients worry about. Heart attack. Stroke. Heart failure. Drug safety. LEGEND synthesizes real-world evidence to determine how different drug classes impact the people who have to choose between them.”
“We did not execute our study to prove one particular drug class was most effective,” Suchard added. “Instead, we used the high-level analytics and best practices developed within OHDSI to study all of these drug classes against each other and openly report on all possible comparisons. Researchers can then interpret specific results in the context of their own research questions.”
The paper also reported that non-dihydropyridine calcium channel blockers proved inferior to the four other first-line antihypertensive drug classes recommended in the 2017 guidelines; other classes included are angiotensin receptor blockers and dihydropyridine calcium channel blockers.
A LEGEND-ary Approach to Observational Science
“LEGEND is a unique, sophisticated approach to using observational data in a way that is reliable, rich and relevant,” Suchard said. “With the availability of existing health data available, we can start to answer important clinical questions in a reproducible manner.”
The LEGEND Hypertension project used state-of-the-art causal methods to address both observed confounding and residual bias. Covering patients from July 1996 to March 2018, the study filled in evidence gaps that were unavailable for the 2017 ACC/AHA guidelines. The RCTs from those guidelines factored approximately 31,000 users of either thiazide diuretics or ACE inhibitors, far fewer than the approximately 3.2 million new-users available in the LEGEND project.
“LEGEND is a novel approach that could transform the way we use real-world evidence in healthcare,” said senior author Patrick Ryan, PhD (Columbia University). “Rather than inefficiently conducting bespoke analyses one-question-one-method-one-database-at-a-time, leaving us vulnerable to various threats to scientific validity, LEGEND provides a systematic framework that can reproducibly generate evidence by applying advanced analytics across a network of disparate databases for a wide array of exposures and outcomes.”
“Not only does LEGEND offer a path to scale to the real needs of the healthcare community,” Ryan added. “it also provides the complementary diagnostics to help us understand how much we can trust the evidence we've produced.”
The paper by Suchard et al. was developed through tools and best practices set by the OHDSI network, a multi-stakeholder, interdisciplinary collaborative to bring out the value of health data through large-scale analytics. OHDSI is an open-science community which, through its central coordinating center at Columbia University, has established an international network of research and observational health databases. OHDSI, which has produced scores of peer-reviewed papers since its 2014 inception, develops open-source analytics tools to generate and disseminate real-world evidence.
Written by Craig Sachson
The UCLA Fielding School of Public Health, founded in 1961, is dedicated to enhancing the public's health by conducting innovative research, training future leaders and health professionals from diverse backgrounds, translating research into policy and practice, and serving our local communities and the communities of the nation and the world. The school has more than 600 students from more than 25 nations engaged in carrying out the vision of building healthy futures in greater Los Angeles, California, the nation and the world.
Faculty Referenced by this Article
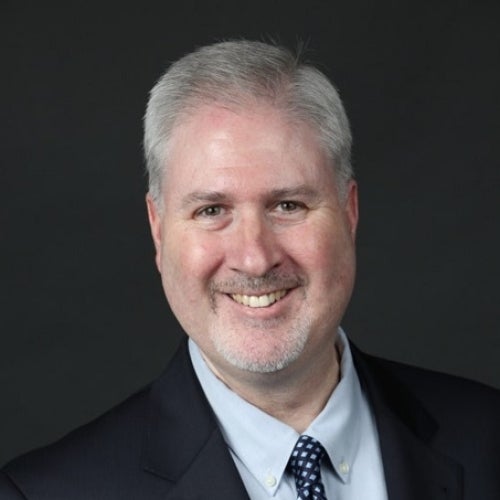
Automated and accessible artificial intelligence methods and software for biomedical data science.